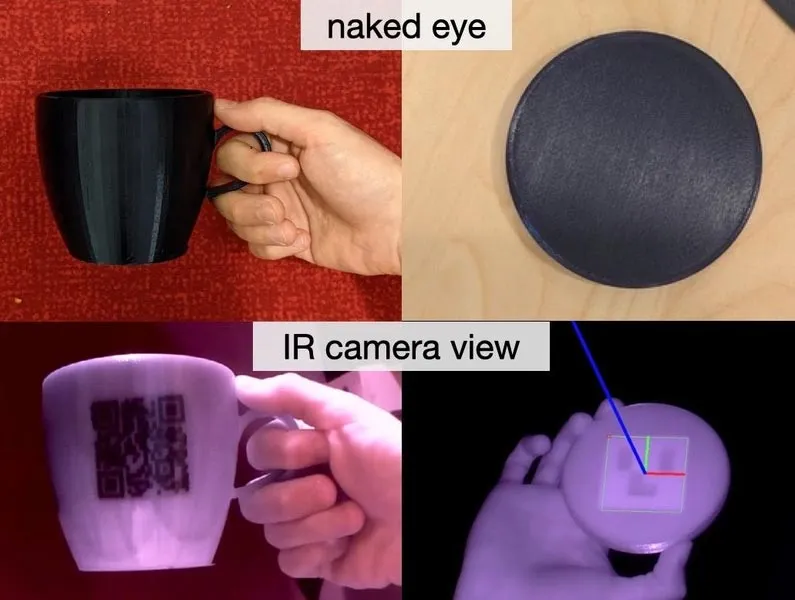
Invisible 3D-Printed Machine-Readable Labels That Identify and Track Objects
ABSTRACT:
Existing approaches for embedding unobtrusive tags inside 3D obgaps inside for the tag’s bits, which appear at a diferent intensity in the infrared image. We built a user interface that facilitates the integration of common tags (QR codes, ArUco markers) with the object geometry to make them 3D printable as InfraredTags. We also developed a low-cost infrared imaging module that augments existing mobile devices and decodes tags using our image processing pipeline. Our evaluation shows that the tags can be detected with little nearinfrared illumination (0.2lux) and from distances as far as 250cm. We demonstrate how our method enables various applications, such as object tracking and embedding metadata for augmented reality and tangible interactions. jects require either complex fabrication or high-cost imaging equipment. We present InfraredTags, which are 2D markers and barcodes imperceptible to the naked eye that can be 3D printed as part of objects, and detected rapidly by low-cost near-infrared cameras. We achieve this by printing objects from an infrared-transmitting flament, which infrared cameras can see through, and by having air
An MIT team develops 3D-printed tags to classify and store data on physical objects.
If you download music online, you can get accompanying information embedded into the digital file that might tell you the name of the song, its genre, the featured artists on a given track, the composer, and the producer. Similarly, if you download a digital photo, you can obtain information that may include the time, date, and location at which the picture was taken. That led Mustafa Doga Dogan to wonder whether engineers could do something similar for physical objects. “That way,” he mused, “we could inform ourselves faster and more reliably while walking around in a store or museum or library.”
The idea, at first, was a bit abstract for Dogan, a 4th-year PhD student in the MIT Department of Electrical Engineering and Computer Science. But his thinking solidified in the latter part of 2020 when he heard about a new smartphone model with a camera that utilizes the infrared (IR) range of the electromagnetic spectrum that the naked eye can’t perceive. IR light, moreover, has a unique ability to see through certain materials that are opaque to visible light. It occurred to Dogan that this feature, in particular, could be useful.
The concept he has since come up with — while working with colleagues at MIT’s Computer Science and Artificial Intelligence Lab (CSAIL) and a research scientist at Facebook — is called InfraredTags. In place of the standard barcodes affixed to products, which may be removed or detached or become otherwise unreadable over time, these tags are unobtrusive (due to the fact that they are invisible) and far more durable, given that they’re embedded within the interior of objects fabricated on standard 3D printers
Last year, Dogan spent a couple of months trying to find a suitable variety of plastic that IR light can pass through. It would have to come in the form of a filament spool specifically designed for 3D printers. After an extensive search, he came across customized plastic filaments made by a small German company that seemed promising. He then used a spectrophotometer at an MIT materials science lab to analyze a sample, where he discovered that it was opaque to visible light but transparent or translucent to IR light — just the properties he was seeking.
The next step was to experiment with techniques for making tags on a printer. One option was to produce the code by carving out tiny air gaps — proxies for zeroes and ones — in a layer of plastic. Another option, assuming an available printer could handle it, would be to use two kinds of plastic, one that transmits IR light and the other — upon which the code is inscribed — that is opaque. The dual material approach is preferable, when possible, because it can provide a clearer contrast and thus could be more easily read with an IR camera.
The tags themselves could consist of familiar barcodes, which present information in a linear, one-dimensional format. Two-dimensional options — such as square QR codes (commonly used, for instance, on return labels) and so-called ArUco (fiducial) markers — can potentially pack more information into the same area. The MIT team has developed a software “user interface” that specifies exactly what the tag should look like and where it should appear within a particular object. Multiple tags could be placed throughout the same object, in fact, making it easy to access information in the event that views from certain angles are obstructed.
“InfraredTags is a really clever, useful, and accessible approach to embedding information into objects,” comments Fraser Anderson, a senior principal research scientist at the Autodesk Technology Center in Toronto, Ontario. “I can easily imagine a future where you can point a standard camera at any object and it would give you information about that object — where it was manufactured, the materials used, or repair instructions — and you wouldn’t even have to search for a barcode.”
Dogan and his collaborators have created several prototypes along these lines, including mugs with bar codes engraved inside the container walls, beneath a 1-millimeter plastic shell, which can be read by IR cameras. They’ve also fabricated a Wi-Fi router prototype with invisible tags that reveal the network name or password, depending on the perspective it’s viewed from. They’ve made a cheap video game controller, shaped like a wheel, that is completely passive, with no electronic components at all. It just has a barcode (ArUco marker) inside. A player simply turns the wheel, clockwise or counterclockwise, and an inexpensive ($20) IR camera can then determine its orientation in space.
In the future, if tags like these become widespread, people could use their cellphones to turn lights on and off, control the volume of a speaker, or regulate the temperature on a thermostat. Dogan and his colleagues are looking into the possibility of adding IR cameras to augmented reality headsets. He imagines walking around a supermarket, someday, wearing such headsets and instantly getting information about the products around him — how many calories are in an individual serving, and what are some recipes for preparing it?
Kaan Aksit, an associate professor of computer science at University College London, sees great potential for this technology. “The labeling and tagging industry is a vast part of our day-to-day lives,” Aksit says. “Everything we buy from grocery stores to pieces to be replaced in our devices (e.g., batteries, circuits, computers, car parts) must be identified and tracked correctly. Doga’s work addresses these issues by providing an invisible tagging system that is mostly protected against the sands of time.” And as futuristic notions like the metaverse become part of our reality, Aksit adds, “Doga’s tagging and labeling mechanism can help us bring a digital copy of items with us as we explore three-dimensional virtual environments.”
The paper, “InfraredTags: Embedding Invisible AR Markers and Barcodes into Objects Using Low-Cost Infrared-Based 3D Printing and Imaging Tools,” (DOI: 10.1145/3491102.3501951) is being presented at the ACM CHI Conference on Human Factors in Computing Systems, in New Orleans this spring, and will be published in the conference proceedings.
Dogan’s coauthors on this paper are Ahmad Taka, Michael Lu, Yunyi Zhu, Akshat Kumar, and Stefanie Mueller of MIT CSAIL; and Aakar Gupta of Facebook Reality Labs in Redmond, Washington. This work was supported by an Alfred P. Sloan Foundation Research Fellowship. The Dynamsoft Corp. provided a free software license that facilitated this research.